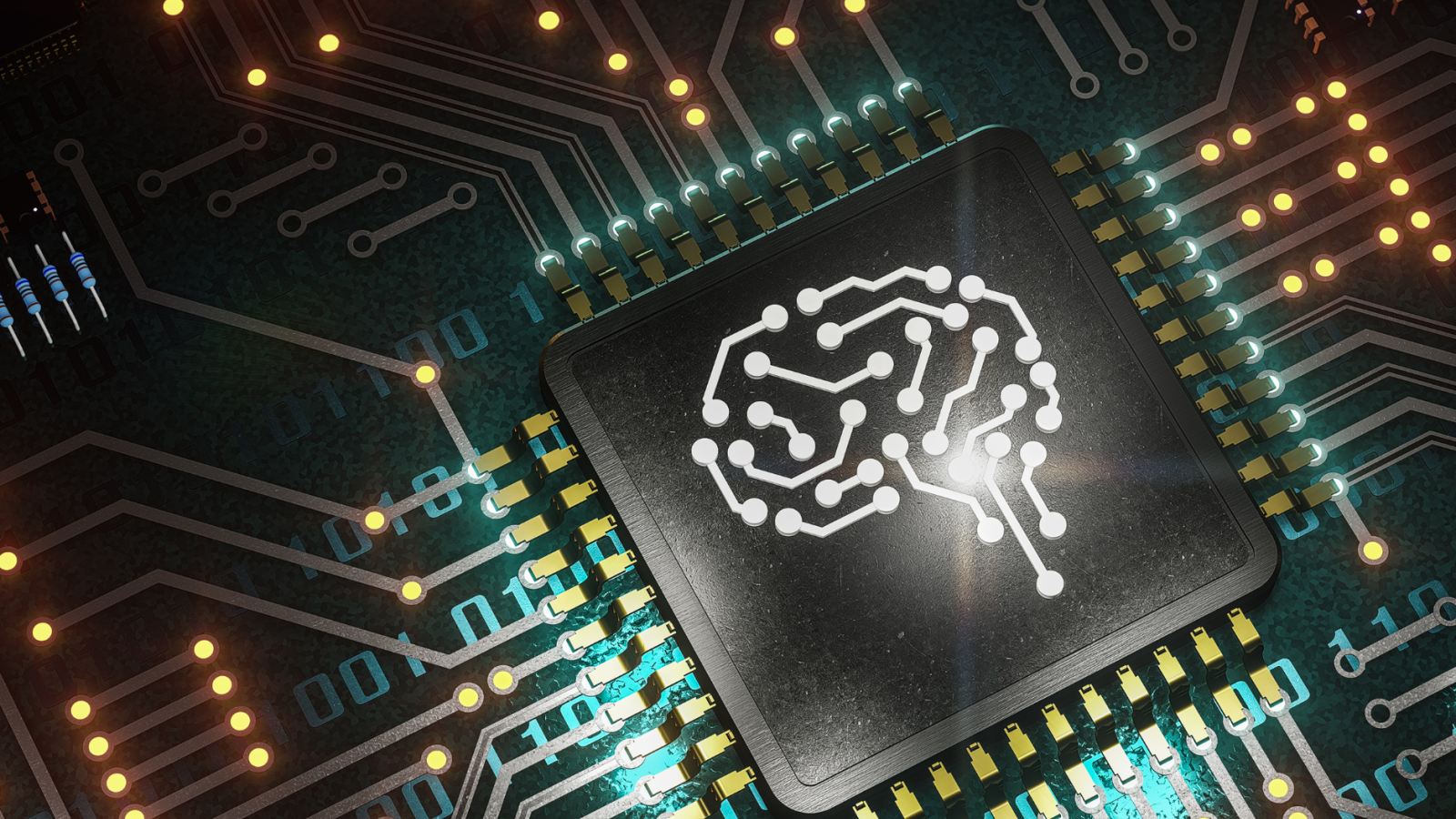
The ECGI blog is kindly supported by
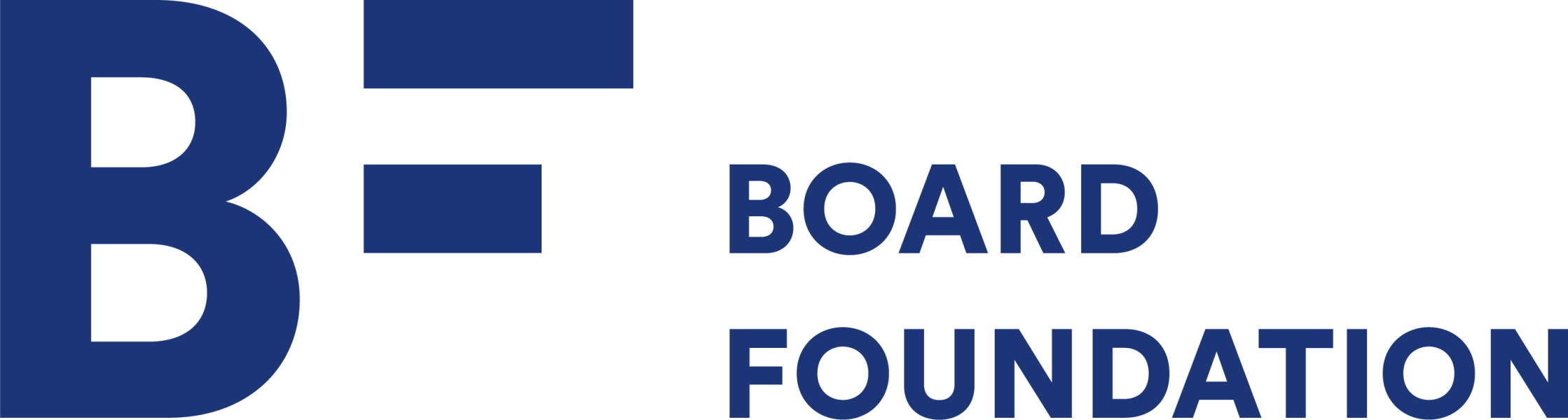
Regulating auditing algorithms: An Asian solution?
Artificial intelligence (AI) is increasingly impacting our society and economy by undergirding, digitizing, and automating important processes across many sectors, such as loan applications, medical diagnoses, hiring decisions, surfacing information, and driving autonomous vehicles. With such important outcomes for individuals, organizations, and society, it is critical that AI can be trusted to make fair and accurate decisions. However, information asymmetries between organizations, consumers and regulators are common, and they are exacerbated by the inherent complexities of algorithmic systems such as machine learning, deep learning, and black box algorithms. Therefore, these information asymmetries can ultimately be exploited by certain organizations, and they can reasonably create skepticism and mistrust on the use of algorithms.
As a response, algorithmic auditing has emerged as a possible solution to address this information asymmetry and create trust by ensuring that a system is reviewed, prior to and during deployment, by a third party with suitable specialist knowledge who can convey information about the impact of the system to other parties. AI audits can take different forms, from checking governance mechanisms, to testing an algorithm’s outputs, to inspecting how AI systems are designed. Audits can be undertaken by external parties appointed by organizations using AI, or by regulators, researchers or other parties carrying out an audit of a system on their own initiative.
As AI becomes more sophisticated and broadly used, algorithmic auditing also involves increasingly complicated ethical, social, and regulatory challenges with different dimensions and implications depending on the sector where the AI is deployed.
While the objectives that inform financial audits, deeply explored in the law and finance literature, may translate to AI in the sense that a financial auditor gathers and inspects evidence to determine whether a company’s practices are free from material misstatement and the company’s financial statements have been prepared according to generally accepted accounting principles, an AI auditor faces more complex challenges including examining design documents, code, and training data to determine whether a company’s algorithms are free from material potentially consequential impact. As AI becomes more sophisticated and broadly used, algorithmic auditing also involves increasingly complicated ethical, social, and regulatory challenges with different dimensions and implications depending on the sector where the AI is deployed. In this context, regulators play a key role in proposing policies to govern the operations, credentials, and impact of the experts conducting AI audits. Additionally, when they act as independent (external) parties, there are no rules governing what – in the context of financial audits – have been named the auditors’ independence puzzle.
The predicament remains daunting, and the nascent ecosystem of external and internal algorithmic auditing is growing in a fragmented manner, without proper scrutiny, guidance, or consistency. The current AI audit landscape still lacks specific rules and standards, and auditors are offering auditing services without assurance of their quality, and the scope and expectations of their review. That can make audits a costly exercise that is not necessarily translated into a higher level of trust and consumer protection. Moreover, it can lead to inconsistencies. One example of the inconsistency that AI audits are plagued with is the apparent international agreement about ensuring fairness in AI implementation. However, when it comes to developing actual evaluations of fairness to audit algorithms, there are numerous statistical definitions of fairness that are often mutually exclusive or that do not match with legal standards of fairness and discrimination. Regulators are expected to propose solutions for this patchwork in the world of AI audits.
Currently, some regulators are showing interest in assessing the performance of AI systems. The European Union has proposed the AI Act, a risk-based approach to AI regulation. The proposed regulatory initiative establishes that for certain systems – High Risk AI systems – an external notified body will be involved in the conformity assessment audit. Likewise, in February 2022, a group of U.S. Senators proposed legislation for an Algorithmic Accountability Act that would have required the Federal Trade Commission to gather reports on algorithms and scrutinize their functions.
Regulators and authorities in Asia are not proposing a regulatory framework for AI but are among the first movers in taking actionable steps towards building AI audit systems.
Nonetheless, these proposals have not been implemented and the principle-based approach of the EU AI Act has not been grounded in specific assessment measures to be implemented in practical use cases. In contrast, regulators and authorities in Asia are not proposing a regulatory framework for AI but are among the first movers in taking actionable steps towards building AI audit systems. These initiatives could shed some light on what regulators in different sectors could focus on for developing an adequate algorithmic auditing ecosystem.
For instance, China’s internet watchdog released some details of how AI systems work inside Chinese tech companies. The Cyberspace Administration of China required the 30 largest domestic tech companies to share AI systems’ information without having to publicly disclose intellectual property. As a result, it is known now that, for example, ByteDance’s algorithm takes likes and dislikes into consideration when serving personalized and targeted content on Douyin, the Chinese version of TikTok. The agency published similar information about companies such as Alibaba and Tencent and required additional information that was not released to the public, including a self-appraisal on the security of the algorithms, the data they collect, whether that encompasses sensitive biometric or identity information, and what data sources are used to train algorithms.
Authorities in Singapore have also developed actionable tools to build a less fragmented AI audit ecosystem. Namely, Singapore’s Infocomm Media Development Authority and the Personal Data Protection Commission (PDPC) launched AI Verify, the world’s first AI Governance Testing Framework and Toolkit for companies that wish to demonstrate responsible AI in an objective and verifiable manner. It verifies the performance of an AI system against the developer’s claims and with respect to the accepted AI ethics principles. The toolkit is a software package that can be downloaded and executed locally in business environments to generate testing reports for engineers and management. Even though AI Verify is not mandatory, 10 companies from different sectors and of different scale, have already tested and provided feedback to the initiative. These companies include Amazon Web Services, DBS Bank, Google, Meta, Microsoft, Singapore Airlines, NCS (Part of Singtel Group)/Land Transport Authority, Standard Chartered Bank, UCARE.AI, and X0PA.AI.
Also in Singapore, the financial regulator, the Monetary Authority of Singapore, worked with technology companies and financial institutions to design an assessment methodology for AI use cases in the financial sector, such as algorithmic credit scoring, that are broadly used in the industry or create risks that should be mitigated as a priority. As part of Veritas, MAS released the first version of an open-source software toolkit that aims to drive financial institutions’ adoption and adherence to AI governance principles. The software enables the automation of metrics assessment and visualization, with plug-ins integrating with financial institutions’ IT systems.
The documented early lessons of these authorities should provide regulators with tools that can assist in providing answers to legal and regulatory challenges regarding the standards applicable to AI systems
These initiatives are the first of its kind globally. The documented early lessons of these authorities should provide regulators with tools that can assist in providing answers to legal and regulatory challenges regarding the standards applicable to AI systems, the scope of auditing systems, the role of auditing systems in compliance, and the translation to ethical principles into actionable and measurable characteristics. These efforts are also pivotal in shaping the AI audit ecosystem as a balance of public and private actors and processes. As such, the role of regulators is crucial for achieving certainty and meaningful AI audits that truly contribute to create trustworthy AI.
--------------------------------
By Nydia Remolina, Assistant Professor of Law, Singapore Management University, Fintech Track Lead and Head of Industry Relations, SMU Centre for AI and Data Governance
This article reflects solely the views and opinions of the authors. The ECGI does not, consistent with its constitutional purpose, have a view or opinion. If you wish to respond to this article, you can submit a blog article or 'letter to the editor' by clicking here.