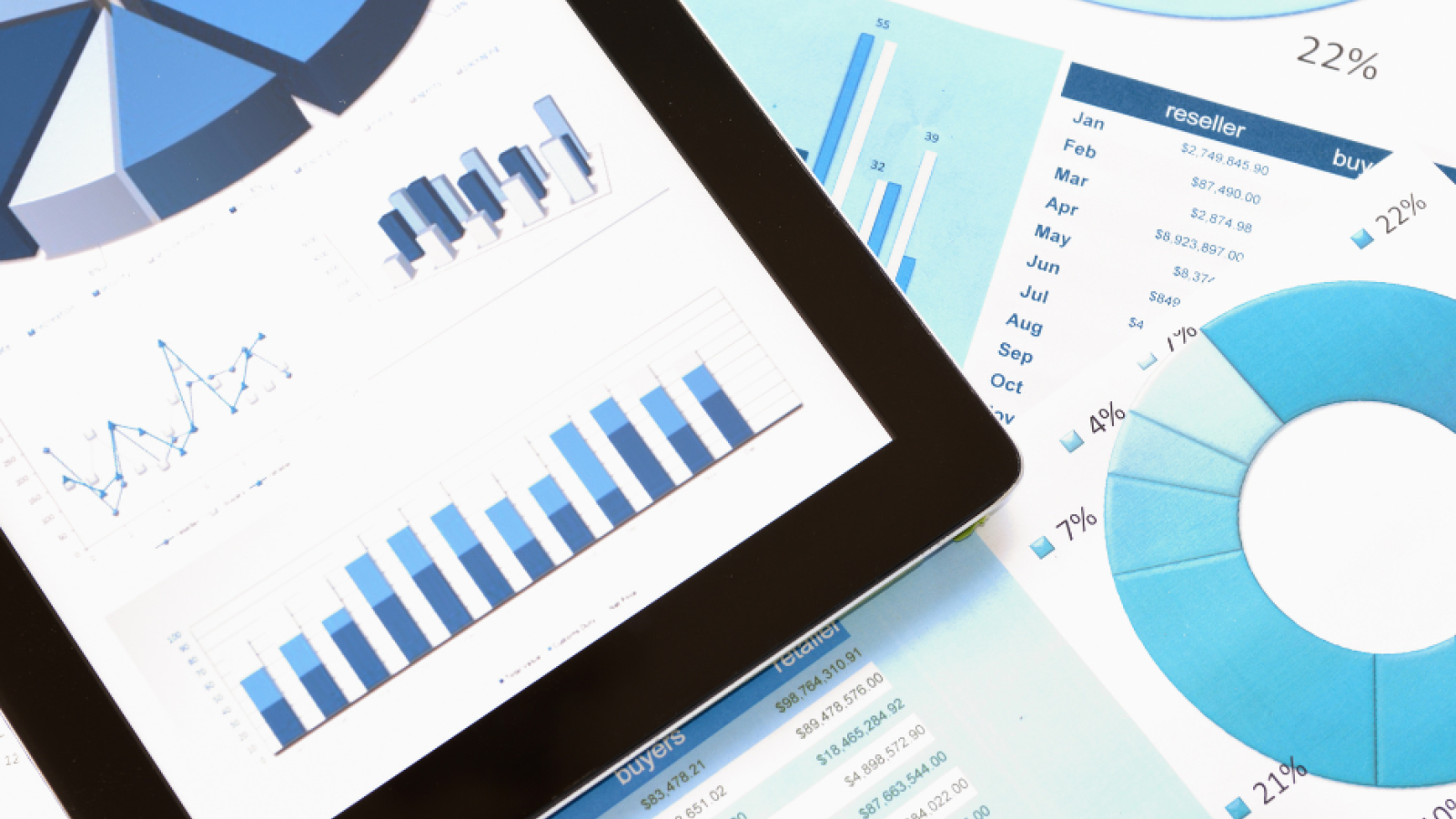
The ECGI blog is kindly supported by
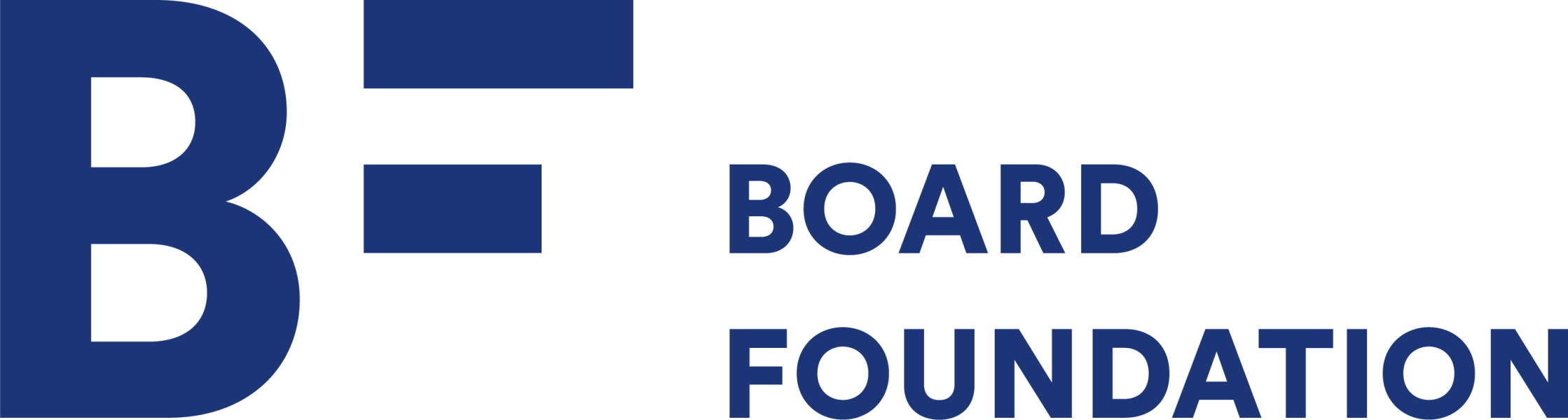
Technology and Governance: Data and Welfare in Credit Markets
Policymakers have implemented many regulations that limit the kinds of data that lenders can use in making lending decisions. Is society better off when data is removed from markets?
Data is an increasingly important topic in corporate and consumer lending. The explosion of data available to screen and score borrowers over the past half century raises important questions about how borrowers are affected by the use of such data.
Moreover, policymakers have implemented many regulations that limit the kinds of data that lenders can use in making lending decisions. Is society better off when data is removed from markets?
Many regulations govern the use of data: for example, the EU's General Data Protection Regulation (GDPR), the FTC's Fair Credit Reporting Act (FCRA), and California Consumer Privacy Act of 2018 (CCPA). Our new paper provides insights into who wins and loses when policymakers prevent markets from using data to make lending decisions.
We construct a framework to quantify the welfare effects of regulatory policies that govern data usage in credit markets. We think of data policy as a form of price discrimination in which a company charges different prices to different consumer groups--similar to a museum offering discounts to students and charging higher prices to non-students. Similarly, data usage in financial markets can be thought of as a kind of third-degree price discrimination.
Should society allow lenders to observe bankruptcy "flags" for previously bankrupt individuals, and set different prices for previously bankrupt and never-bankrupt borrowers?
Our study develops an example to illustrate this. Consumers who've been bankrupt in the past tend to be at higher risk of defaulting on a loan than consumers who have never been bankrupt. When lenders can tell formally bankrupt consumers apart from non-bankrupt consumers, they will set higher prices (i.e., higher interest rates) for previously bankrupt consumers, and lower prices for never-bankrupt consumers consistent with each consumer’s likelihood of repaying the loan. Should society allow lenders to observe bankruptcy "flags" for previously bankrupt individuals, and set different prices for previously bankrupt and never-bankrupt borrowers?
Now suppose that a policymaker wants to help formerly bankrupt consumers. Since redistributing cash directly is often difficult, we might think of deleting bankruptcy information as an alternative: we redistribute through subsidized prices in credit markets by preventing lenders from using this information. In fact, the FCRA requires that flags indicating the occurrence of consumer bankruptcy be removed after seven (ten) years for a Chapter 13 (Chapter 7) bankruptcy. In such a case, the lender will not be able to distinguish formerly bankrupt borrowers and those that have never declared bankruptcy.
This loss of information has two effects on borrowers. First, the removal of bankruptcy information effectively transfers surplus from never-bankrupt consumers to previously bankrupt consumers. Second, we show that society as a whole is better off with more data: social welfare decreases since the prices no longer reflect the risk of the borrowers. This contrasts with the setting in which lenders have access to the borrower bankruptcy history and they set the prices to reflect the cost of lending to each borrower type, thus making credit allocation more efficient.
If the policymaker is interested in redistributing wealth to previously bankrupt consumers, preventing the use of bankruptcy data helps to achieve this.
An important finding of our study is that the transfer effects of data availability in the case of bankruptcy data availability tends to be large relative to the welfare effects. That is, the social welfare gains of having the additional information is small relative to the size of the transfer between the two borrower types. Thus, if the policymaker is interested in redistributing wealth to previously bankrupt consumers, preventing the use of bankruptcy data helps to achieve this.
Given that there are many ways in which a policymaker can transfer wealth to segments of the population, it is important to consider the cost of such a transfer. How much welfare is lost in this setting?
When we apply our framework to the US auto lending market and find that for every dollar transferred to previously bankrupt consumers only 3 cents of social surplus are lost. This implies that the removal of bankruptcy information is a fairly efficient way to transfer wealth to previously bankrupt consumers.
While this example is focused on a particular consumer data setting, our framework can be easily applied to many corporate and consumer lending environments. The framework is simple to implement empirically: to measure the welfare and transfer effects of data availability or policy change, one only needs to observe the pre- and post-data prices (interest rates in our earlier example) and quantities (loan size in the earlier example) for each consumer group.
So, in summary, our paper introduces a framework for thinking quantitatively about the welfare and distributional effects of data policy, which can be taken to data pretty easily. We hope others use this method to analyze the welfare effects that data policies have in other settings.
--------------------------------
By Anthony Lee Zhang, University of Chicago - Booth School of Business, Constantine Yannelis, University of Chicago - Booth School of Business & Mark Jansen, University of Utah
This article reflects solely the views and opinions of the authors. The ECGI does not, consistent with its constitutional purpose, have a view or opinion. If you wish to respond to this article, you can submit a blog article or 'letter to the editor' by clicking here.