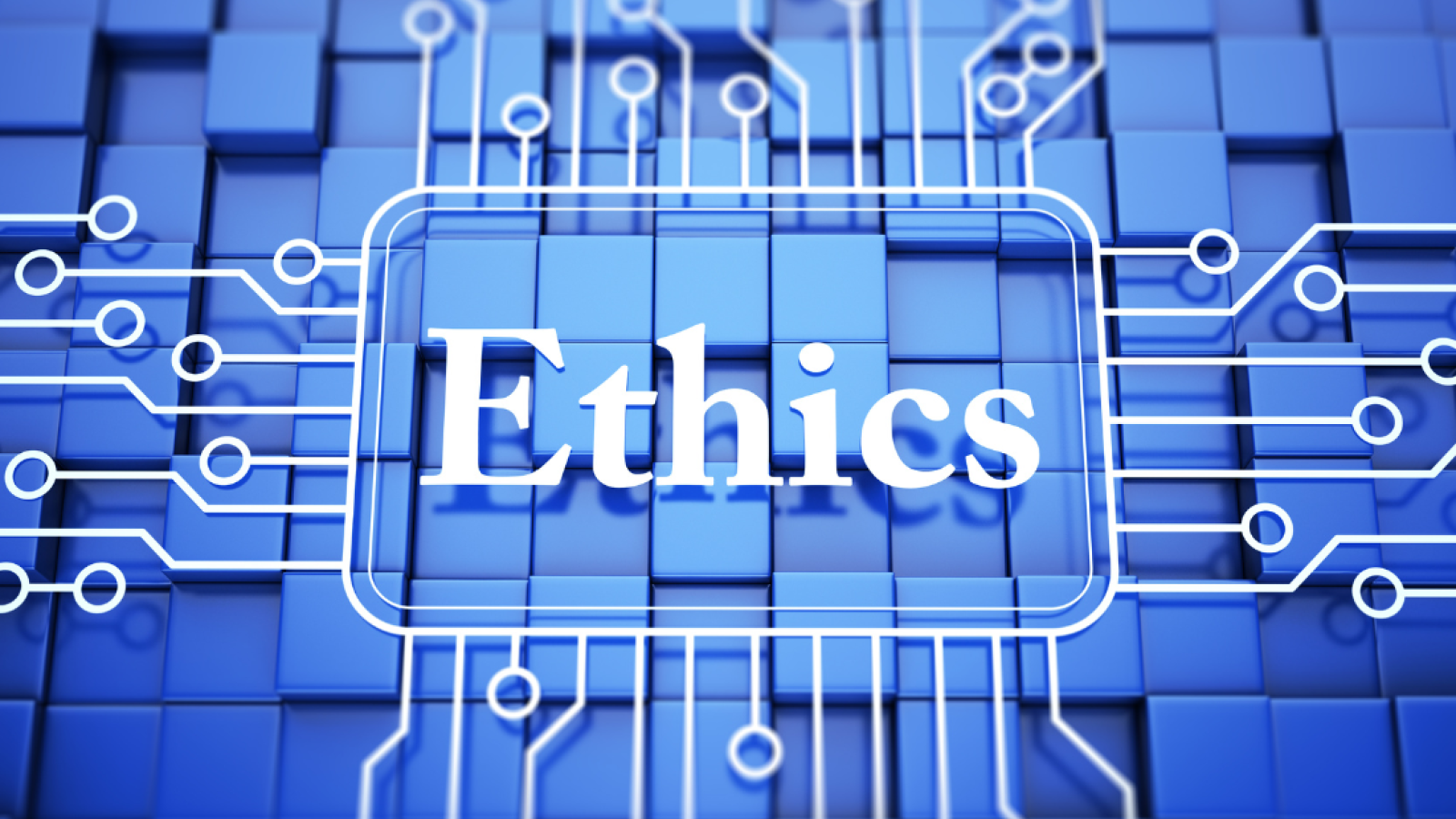
The ECGI blog is kindly supported by
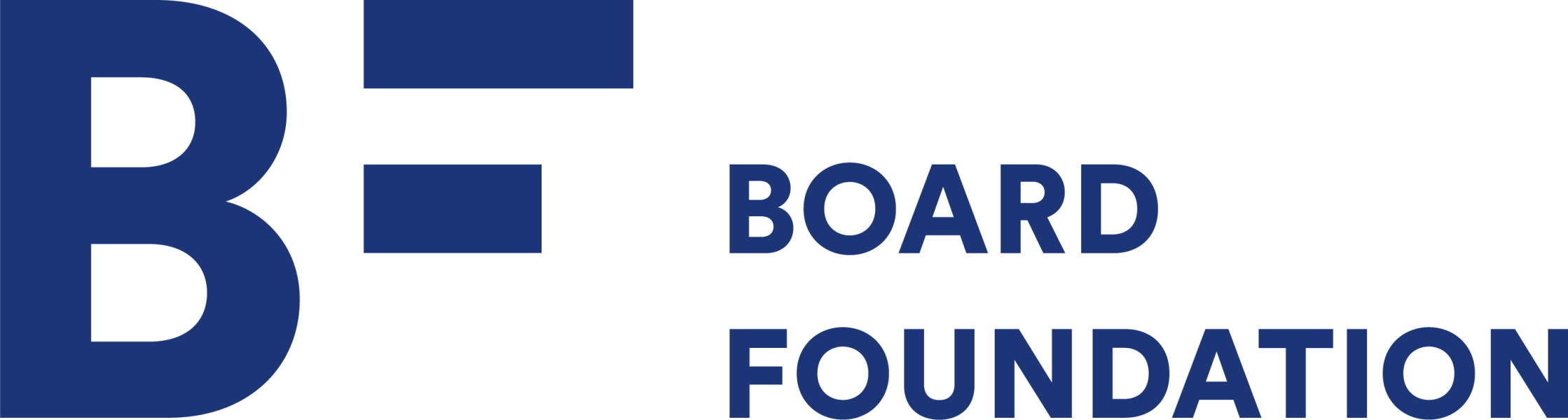
The rise of alternative data: AI governance and ethical challenges
It has been said that ‘data is the new oil’. It was to indicate data is an asset, a powerful resource allowing organisations to optimise their business models and increase profits. But it’s beginning to look like the analogy is also true in relation to risk and liability linked to collecting and using data: the last few months in Australia looked like the BP Deepwater Horizon and Exxon Valdez disasters combined, with Optus (second largest telecom company in Australia) and Medibank (one of the largest Australian private health insurers) data breaches. Up to 20 million people – current and past customers – are reported to have been affected. It provoked a bit of a knee-jerk reaction from the government, which quickly proposed legislation increasing penalties for data breaches and enhancing regulators enforcement powers.
Cybersecurity breaches, while onerous for both companies and customers affected, are far from being the only risk for organisations involved in the data economy. While governments and consumers tend to focus on problems such as identity theft (no doubt an important issue), new legal and ethical challenges arise from the increasingly ubiquitous collection and use of alternative data. Such non-traditional, new streams of data used can include, for example, social media, internet browsing history, smartphone apps, location history, customer loyalty schemes, fitness trackers, smart home devices and so on.
Consumers are often unaware of the ongoing omnipresent alternative data collection, aggregation and combining.
Powerful data analytics tools, enabled by AI and related technologies, such as machine learning, make it possible to analyse and learn from all that data, generating inferences and predicting trends that would be otherwise unobservable to humans. And while these insights may be of high commercial value, they bring about legal and ethical challenges. Consumers are often unaware of the ongoing omnipresent alternative data collection, aggregation and combining. Datasets which are supposed to be de-identified or anonymised, are often easily re-identifiable. AI models used to analyse the data are often opaque black boxes, which makes explaining and potentially challenging of their decisions difficult. The predictions of the models may be inaccurate, adversely affecting often the most vulnerable consumers. Not to mention the well-described problem of algorithmic discrimination and bias perpetuated by the models and embedded within the data they are fed.
Still, the discourse about alternative data is mainly that of benefit and opportunity – a necessary component of fostering AI innovation endorsed by companies and governments. McKinsey consulting firm hails ‘harnessing the power of external data’ noting how: ‘few organizations take full advantage of data generated outside their walls. A well-structured plan for using external data can provide a competitive edge.’
Companies boast how AI insights allow them to offer personalised services, ‘tailored’ to individual consumer’s needs. Policymakers also promote ‘innovation’, and encourage data collection, for example through open banking schemes. The aim of open banking is to give consumers the ability to direct companies that hold financial data about themselves to make it available to financial (or other) companies of the consumer’s choice. In practice, it makes it possible for organisations to get access to consumers’ information they could never get from a consumer directly, such as for example their transaction data for the past 10 years.
Financial industry is in particular keen to take advantage of alternative data. ‘All data is credit data, we just don’t know how to use it yet’ is a famous statement summing up the industry thinking. This in turn means that firms are eager to collect any and all data: after all, it may signal the value of the client, improve business processes, and it’s encouraged by industry consultants and even legislators.
Failing to comply with privacy and data protection rules attracts investigation and penalties
However, it looks like a reckoning is coming. Consumers are becoming increasingly aware of data surveillance and dodgy data practices. Reputation risk is becoming something the organisations need to start caring about. Failing to comply with privacy and data protection rules attracts investigation (see for example CHOICE’s work on Australian retailers using facial recognition tech) and penalties, as the Clearview AI case showed. Public opinion pressure is mounting on the policymakers to regulate the use of AI models and people’s data.
Yet it still looks like many organisations believe hiding their data practices behind unclear or misleading privacy policies is the way to go. It should go without saying that it’s not adequate risk management, but quite the opposite: it’s potentially illegal, certainly unethical, and exposing companies to risk.
Responsible, ethical and transparent AI and data governance should be key to anticipate and prevent the risks. ‘Machinewashing’, where companies try to convince stakeholders and regulators that their internal AI and data governance operates in line with human and societal values, while in reality they engage in unethical or even illegal data and AI practices, won’t cut it. Stricter enforcement and new data and AI regulation (such as the EU’s AI Act) is coming, and it will make transparent and ethical AI and data governance a necessity for organisations. Alternative data, as much as is an asset and a resource, is also a liability and a risk that needs to be addressed. The sooner, the better.
-------------------------------------------------------------------------------------
By Dr Zofia Bednarz, Lecturer in Commercial and Corporate Law, University of Sydney; Associate Investigator, Australian Research Council Centre of Excellence for Automated Decision-Making and Society
The ECGI does not, consistent with its constitutional purpose, have a view or opinion. If you wish to respond to this article, you can submit a blog article or 'letter to the editor' by clicking here.